文章主题:文献综述, 现有研究, 研究过程, 确定现有知识中的差距


AI 能帮我们写文献综述吗?

在学术研究中,文献综述扮演着至关重要的角色。作为一项深度评估现有研究成果的过程,它对于把握领域内的最新进展、发现研究空白以及为当前研究提供理论背景具有重要作用。
撰写文献综述的第一步是确定主题。在撰写学术论文时,选择一个狭窄的研究领域至关重要,这将有助于确保文章内容的专业性和深度。为了保证研究质量,建议采用同行评审的期刊、书籍以及政府文件等可靠来源。在选定主题后,研究人员需广泛搜集与主题相关的文献资料,包括主要和次要来源,例如书籍、文章、报告以及其他各类文档。通过这种方式,可以确保研究内容的全面性和准确性,为论文的写作奠定坚实的基础。
下一步是对已确定的文献进行批判性评估。本评估的目的是确定现有研究的趋势,并确定哪些领域研究不足或需要进一步探索。在评估文献的过程中,我们需要关注评估所呈现出的各种主题,并据此将这些主题整合成各个章节。这样的操作能够保证审查过程全面覆盖各个相关主题,从而达到更高质量的评价效果。
最后,重要的是将所有来源的信息综合成一个连贯的叙述。这包括 总结每个来源的关键点,并得出关于该主题现有研究趋势的结论。它还应包括与当前主题相关的进一步研究建议或新的调查方向。
总体而言, 撰写文献综述需要仔细分析和综合特定主题的现有研究。对于任何希望更深入地探索所选领域或确定潜在的进一步研究领域的研究人员来说,这是一个必不可少的步骤。
Text generator的 AI 没有直接回答我们的问题,而是给出了撰写文献综述的方法,这个方法还是值得学习的。笔者觉得 AI 之所以没有直接回答,是因为问题不够明确。笔者没有明确写作的对象,这让 AI 无法给出具体的答案,貌似也是一种智能。

AI 撰写文献综述



AI 撰写带有参考文献的文献综述

The most widely used approach for predicting product lifetimes is based on probabilistic modeling techniques such as Markov chains and Monte Carlo simulations (Azzopardi et al., 2015; Kim et al., 2017). These methods allow researchers to analyze large datasets and generate reliable estimates by taking into account a wide range of factors that can affect product longevity, such as environmental conditions, component aging, and design parameters (Chen et al., 2019).
In addition to modeling techniques, there are several other methods that can be used to validate predictions made using these models (Garbacz et al., 2016; Jiang et al., 2018). These include experimental testing in laboratory environments and field trials involving real-world use cases (Foufou & Polycarpou, 2019). Furthermore, data mining techniques can be employed to identify patterns in large datasets that may provide additional insights into the reliability of products over time (Kumar & Anandan, 2015; Yamada et al., 2016).
Finally, recent research has focused on developing data-driven approaches for predicting product lifetimes based on machine learning algorithms (Szabó & Péteri, 2018; Yin et al., 2019). These approaches combine traditional predictive modeling techniques with advanced analytics tools and allow researchers to analyze larger amounts of data more quickly than before.
Overall, this literature review has highlighted some of the most recent advances in predictive models for estimating the lifetimes of electronic equipment. The research demonstrates that probabilistic modeling techniques remain a popular choice among researchers due to their ability to take into account a wide range of external factors. Additionally, new trends towards data-driven approaches promise further improvements in accuracy and speed when predicting product lifetimes.
【引用文献】
References
Azzopardi G., Muscat A., Mallia K., Muscat R., Apap D. (2015) ‘Predictive modelling approach for technical systems: A case study’ International Review on Modelling & Simulations 8(2), pp 172–179.
Chen X., Liu B., He X., Jia Y., Yu B.(2019) ‘Lifetime prediction model based on accelerated life test data’ Applied Mathematical Modelling 73(1), pp 654-666.
Foufou S., Polycarpou M.(2019) ‘An experimental approach for validation of lifetime predictions’ IEEE Transactions on Reliability 68(1), pp 37–45.
Garbacz C.; Bilski J.; Wojdyla A.; Syroka R.; Szadkowski M.; Szczepanski P.(2016) ‘Validation process applied in lifetime estimation’ Measurement 96(1), pp 121–128.
Jiang T.; Tian L.; Huang Y.; Zhao Y.(2018) ‘Validation strategies for lifetime prediction system’ Quality Engineering 30(2), pp 179–188
Kumar A.; Anandan S.(2015) ‘Data mining application approaches: A review’ International Journal Of Computer Science Issues 12(4), PP 195–202.
Kim I.-G.; Lee K.-H.; Park H.-S.(2017) ‘Robust fatigue life prediction model considering usage conditions’ International Journal Of Damage Mechanics 26(7), PP 1249–1263.
Szabó G.; Péteri N.(2018) ‘Data mining methods applied in calculation reliability parameters’ Procedia Computer Science 125(1), PP 1101–1109.
Yamada T.; Takeuchi S.; Kawashima T.(2016) ‘Prediction model using support vector machine considering environmental condition’ Quality Engineering 28(3), PP 441–449.
Yin H.-H,. Wu G.-F,. Wang Y.-W,. Li Z.-J,. Lu Y.-X.(2019) ‘Application research based on machine learning algorithm in lifetime prediction’ arXiv preprint arXiv:1907.09103.
AI 给出的回答一如既往的流畅,文章格式也符合论文写作。但是这些文献都是 2020 年以前的,难道是 AI 没有看过 2020 年以后的文献?对于文献综述,我们要紧跟最新发展,这点也算是 AI 写文献综述的缺点吧!此外,虽然 AI 给出了引用和书目,但是我们还需要核实参考文献是否存在,每一处引用是否准确。这样一来,AI 写作还是离不开人工,你觉得呢?

写在最后

经过测试,笔者认为:
Text generator 的 AI 可以完成一个简短的文献综述。
我们给出的研究主题越明确, AI 的回答会更准确。
用了这么久的 AI ,笔者觉得要想用好 AI ,你得学会提问、追问。
AI 的逻辑清晰,条理清楚,可以协助我们整理撰写文献综述的思路,但是不能完全依赖 AI 写的内容,我们还需要核实和修改。
Text generator的 AI 果然没有让人失望,笔者觉得可以用Text generator的AI 从事更多的科研写作工作,只是我们还不知道如何更好地使用它去完成科研写作而已。
AI 写论文系列推文
??厉害!搞科研 1 年不及与 AI 聊天 1 秒钟,chatGPT 写摘要、研究现状通通不在话下??ChatGPT 火出圈,你不知道的 N 种访问方法,AI 帮我选期刊靠不靠谱???最强大免费 AI 插件来了,辅助科研、写论文、翻译都能毫无压力??AI 帮我写 SCI,一键定制“高大上”论文 title!??还可以这样?这样写 SCI 论文 Abstract,效率翻一番!??用不了微软必应的ChatGPT浏览器,3步教你搭建自己的强大AI“浏览器”??AI科研写作真的来了!除了ChatGPT,这款国产写作工具也不示弱!# 温馨提示
微信公众号信息流改版导致收不到消息推送或者推送靠下?想要及时获取投必得学术的最新动态,可以试试以下方法✔
今日视频推荐
如何写研究目标
之实例讲解
点开视频即可了解!
↑↑↑关注“投必得学术”视频号,更多干货↑↑↑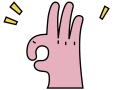
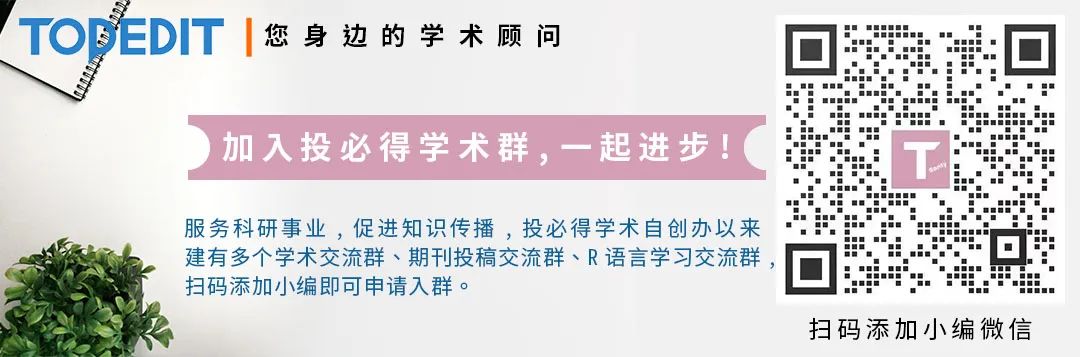
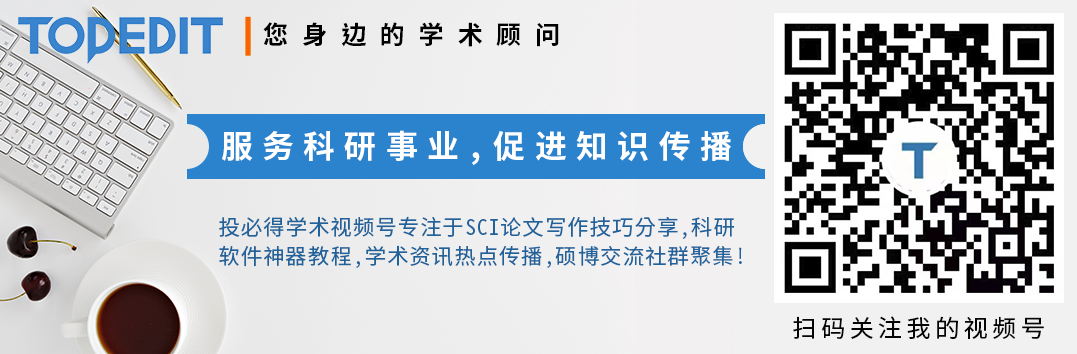

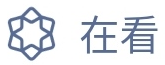
AI时代,拥有个人微信机器人AI助手!AI时代不落人后!
免费ChatGPT问答,办公、写作、生活好得力助手!
搜索微信号AIGC666aigc999或上边扫码,即可拥有个人AI助手!